Helping hands
Finding answers to the really big questions no longer depends on human ingenuity alone: machine science is enabling scientists to go further faster than ever before
First there was Newton, then Einstein. But when it comes to next steps in our understanding of how the world around us actually works, it’s less a case of ‘who’ and more about ‘what’. Welcome to the new machine age.
Deep in the heart of the Centre for Mathematical Sciences, Dr Miles Cranmer is working on, and with, a new dimension in science – a neural network or machine scientist, a series of algorithms that aim to mimic how the human brain works. It’s a sort of Sir Isaac Newton for the AI era.
The network is trained by feeding it data to make it better at its purpose – in the same way that the hugely popular AI chatbot ChatGPT has been trained on millions of pages of text from books, newspaper articles and web pages. But rather than building a way for computers to mimic human speech, for example, Cranmer’s machine scientist has a loftier goal – to become the next Newton, Curie, Einstein or Hawking.
I became interested in machine learning because I started thinking about the pace of scientific discovery
“I became interested in machine learning because I started thinking about the pace of scientific discovery,” says Cranmer, who grew up near Toronto and studied undergraduate physics at McGill University in Quebec. “I remember reading an interview with someone talking about how, in physics, there can be generations before we solve specific problems. That, to me, was very frustrating to hear, but it made me think: ‘How can we accelerate the pace?’” Cranmer realised that machine learning might be able to help, because its analytical power scales exponentially with computing power – which has been growing exponentially for 50 years.
Machine learning
As the machine learning model gets trained, the outputs of the various algorithms are tweaked or weighted. And as the outputs get closer to the actual data, the artificial brain closes in on the best model for that data, something very difficult for the average person to do. This gives the machine scientists a huge advantage, says Cranmer. “The human brain is not like that.”

Take the solar system, and specifically our understanding of the motion of planetary bodies within it. While science dating back to Johannes Kepler and Newton can accurately predict the behaviour of planetary systems with two bodies, adding more bodies makes those systems chaotic and harder to model accurately far into the future, because of the complex instabilities introduced by the gravitational tugs between the growing number of bodies (as fans of the novel The Three-Body Problem, now a Netflix hit series, will know).
Under Cranmer’s supervision, however, the machine scientist is fed tens of thousands of simulated planetary systems. The data is crunched on a single graphics processing unit – the same sort of chip that sits in high-end gaming PCs. And the result? A simple algorithm for planetary systems that provides 10 times greater accuracy than existing equations based on chaos theory.
What machine science allows us to do is crunch large scientific datasets and discover empirical equations that concisely describe the data, without having to work out the theoretical basis in advance.
“What machine science allows us to do is crunch large scientific datasets and discover empirical equations that concisely describe the data, without having to work out the theoretical basis in advance,” says Cranmer. This, he says, is an inversion of the usual process where a theorist comes up with a plausible description of the universe and then experimentalists carry out research to confirm or challenge the data (which is how the existence of the Higgs Boson was proven, for example).
There are precedents for such work. Newton’s law of universal gravitation worked very well for 250 years in predicting the movement of planets. When Albert Einstein came along with his theories of gravitation, scientists realised that while Newton’s equations were good, they only told part of the story. Einstein’s theories boiled down to Newton’s equations in most everyday situations, but Einstein’s tweaks explained phenomena that Newton’s laws could not.
Datasets and equations
But in Cranmer’s world, the machine scientist starts with a simple equation – perhaps the equation of a straight line, the simplest form of equation with the lowest number of parameters. Then the machine starts making small modifications, adding in extra parameters until the equation matches the real-world data as closely as possible.
Given that any set of data can be recreated by a suitably complex equation – the basis of a branch of mathematics called Fourier analysis – the challenge for Cranmer is to ensure that the equation the machine scientist spits out actually represents a real-world physical process. “We are trying to optimise both accuracy and sparsity,” he says.
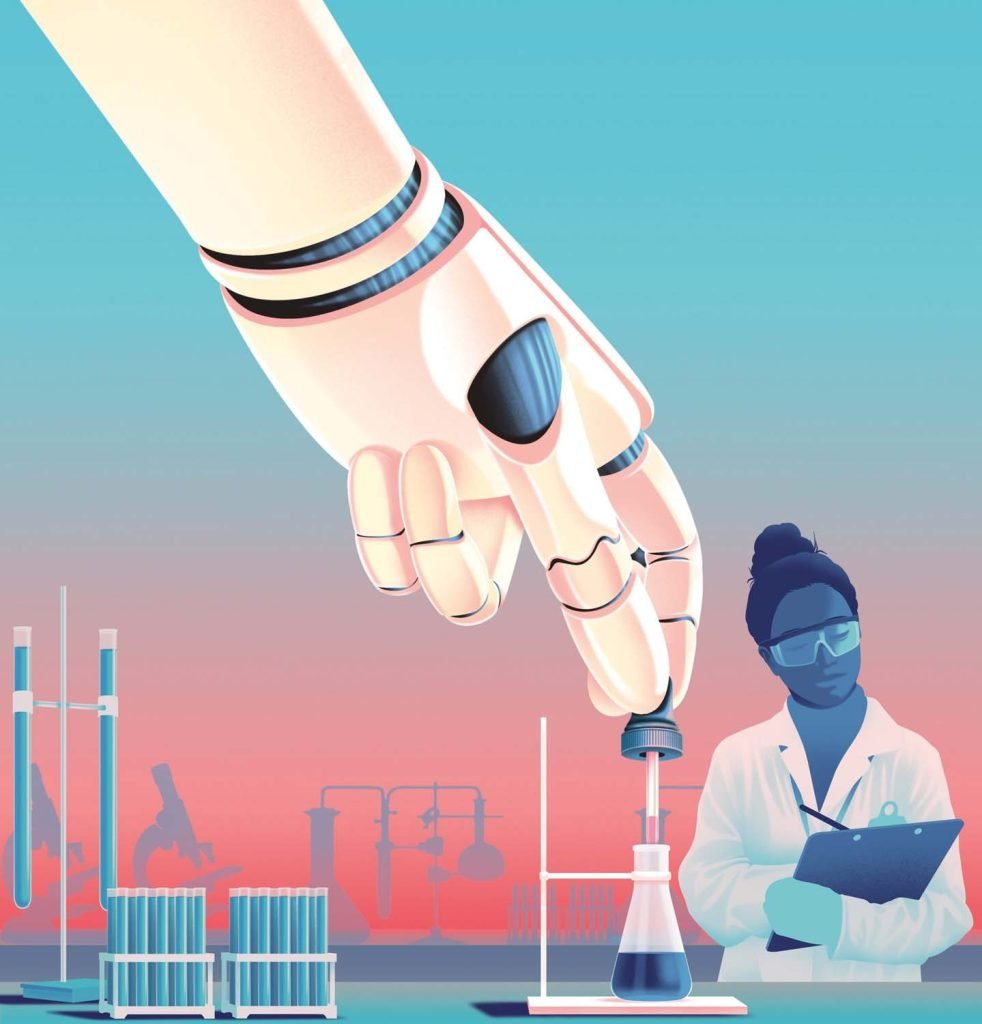
By getting the machine scientist to favour the sparsest equations possible – those with a minimum of parameters and using simple mathematical operators – he hopes it may mirror some underlying physical reality that can be gleaned from looking at the equation. After all, Einstein’s E=mc2 is a relatively simple equation that contained a huge number of new ideas.
The next step for Cranmer, in collaboration with NYU and the Flatiron Institute in New York, is to create something with even grander goals – to build a general AI tool that is multi-disciplinary, learning general models for science that work across many fields – thus accelerating discovery of new scientific frameworks themselves. Rather like generative AI tools such as ChatGPT, the team are now working on creating an all-purpose machine scientist based on what is called a foundation model.
However, unlike ChatGPT, this foundation model will be trained on vast amounts of purely scientific data. It is a fundamental shift in how machine learning is used. “The reason we think building foundation models for science will work is because, in science – as in language, images and videos, for example – we have these universal concepts across different fields,” says Cranmer. “If you zoom out and look at all of science, there are concepts like causality and conservation laws that are shared between disciplines.”
The datasets you use do not need to be closely related to the science you care about
Cranmer believes that applying ideas from one area of science to another could lead to new and faster discoveries. “It’s basically trying to connect different fields of science that we’ve never really had the chance to understand, because you need someone who is an expert in two areas of science to realise the connections,” he says. Cranmer’s all-purpose machine scientist will be called Polymath 1, and is set to be released later this year. The type of data Polymath 1 is being trained on includes the reaction diffusion equation, the compressible flow equations and the Navier-Stokes equation for incompressible fluids, along with many others.
“One thing that is really interesting is that the datasets you use do not need to be closely related to the science you care about,” says Cranmer. “It turns out it doesn’t really need to be related at all. The model will still pick up general concepts from whatever data you feed it. We have even found that machine scientists trained on a dataset of cat videos does better at finding these connections in science. It’s incredible. Cat videos are not related to the movement of fluids, but it is learning how cats move and the physics of that.”
Polymath 1 will be opened up to all scientists, in the hope that they will be able to explore interesting new applications. “People can then start downloading it and fine-tuning it to different tasks,” he says.
Does Cranmer think his machine scientist will replace human ones? Simple answer – no. “Polymath 1 is a tool scientists will use to accelerate the discovery process. The scientists are still key. Even if we can predict something with 100 per cent accuracy, I don’t think scientists would be satisfied. Fundamentally, humans will always want to understand as well as predict the universe.”